Hemberg Group
Quantitative models of gene expression
Archive Page
This page is maintained as a historical record and is no longer being updated.
The Hemberg Group moved to the Evergrande Center for Immunologic Diseases in February 2021. https://evergrande.hms.harvard.edu/home
Recent technological advances have made it possible to analyze gene expression and other related properties in a high-throughput manner, and this has resulted in a wealth of data. However, the experimental data is typically large, high-dimensional and noisy. We are interested in developing computational methods that will make it possible to extract as much information as possible from the data.
Some of the ongoing research projects are:
- Inference of gene regulatory networks from single-cell RNA-seq data. Thanks to extensive annotation efforts, we have an almost complete catalogue of protein coding genes in humans and model organisms. Much less is known about how genes interact. To infer a network, one must have expression data from multiple conditions, e.g. mutants or a time-series. Due to the high levels of noise in the data and the limited number of conditions, existing methods for bulk RNA-seq have a limited ability to detect causal regulatory relations. With single-cell RNA-seq data, a more powerful approach is possible since each cell can be considered as an individual replicate. Knowing which genes interact is also key for understanding development and many diseases such as cancer and autism.
- Identification of the molecular mechanisms involved in transgenerational epigenetic inheritance. Together with the Miska lab, we are studying C. elegans to learn more about how gene expression profiles can be stably inherited. Several lines of evidence have suggested the existence of such effects, but no mechanism has been identified for endogenous genes. The short generation time and the small genome makes C. elegans a powerful model system for investigating this phenomenon.
- Identification and characterization of non-canonical secondary structures in DNA. Mutations outside of coding regions remain poorly understood and their importance in cancer and other diseases is unknown. We are investigation non-coding mutations from cancer samples to find out if the disruption of secondary DNA structures could play an important role.
- Virtual Reality technology for visualizing genomic data. We are collaborating with HammerheadVR, a leading VR development studio to develop a novel genome browser for Virtual Reality technologies.
Previous team members
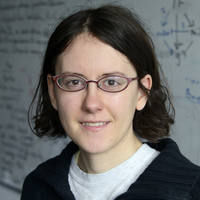
Tallulah S. Andrews
Postdoctoral Fellow
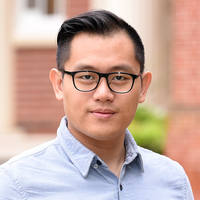
Dr Jimmy Tsz Hang Lee
Postdoctoral Fellow
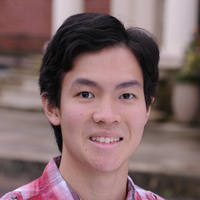
Nicholas Lee
PhD Student
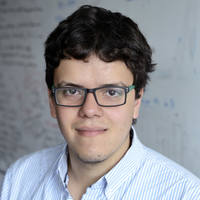
Guillermo Parada
PhD Student
Associated research
Related groups
Programmes and Facilities
Partners
We are interested in working in close collaboration with experimentalists as it provides with direct access to the people who generated data and have a deep understanding of the underlying biology. This approach makes it easier for us to develop mathematical models and it also provides us with a better understanding of what type of computational tools are needed.Some of the people that we have worked with in the past or are currently working with are listed below.
External
Cristophe Lancrin
External
Jesse Gray
External
Tony Kouzarides
External
Eric Miska
External
Mauricio Barahona
External
Gabriel Kreiman
External
Judith Steen
External
Yingxi Lin
External
Jan Pruszak
External
Azad Bonni
External
Michael Greenberg
External
Una-May O'Reilly
External
Naman Jain
External
Steve Jelley
External
Harrison Gabel
External
Meri Huch
External