ADaM
ADaM (Adaptive Daisy Model): an R package for discriminating between core fitness and context-specific fitness genes in large-scale gene essentiality datasets.
The Adaptive Daisy Model (ADaM) package implements a semi-supervised algorithm for computing a fuzzy-intersection of non-fuzzy sets by adaptively determining the minimal number of sets to which an element should belong in order to be a member of the fuzzy-intersection (the membership threshold).
This threshold maximises the deviance from expectation of the cardinality of the resulting fuzzy-intersection, as well as the coverage of predefined elements.
This method can be used to identify the minimal number of cell lines from a given tissue in which the inactivation of a gene (for example via CRISPR-Cas9 targeting) should exert a reduction of viabilty (or fitness effect) in order for that gene to be considered a core-fitness essential gene for the tissue under consideration.
This method is used to discriminate between core-fitness and context-specific essential genes in a study describing a large scale genome-wide CRISPR-Cas9 pooled drop-out screening [1] (a detailed description of the algorithm is included in the Supplemental Information of [1]).
ADaM was inspired by the Daisy Model method introduced in [2]
References:
[1] Behan FM & Iorio F & Picco G et al., In press.
[2] Hart T et al., High-Resolution CRISPR Screens Reveal Fitness Genes and Genotype-Specific Cancer Liabilities. Cell. 2015;163:1515–26.
Downloads
Download from: https://github.com/francescojm/ADAM
Contact
If you need help or have any queries, please contact us using the details below.
Sanger Institute Contributors
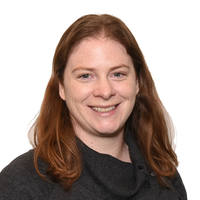
Dr Clare Pacini
Principal Bioinformatician
Previous contributors
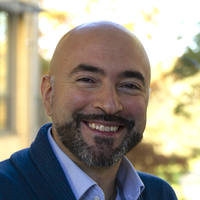
Dr Francesco Iorio
Principal Staff Scientist