METACARPA
METACARPA performs scalable meta-analysis between genetic association studies, both effect-size based and p-value based, while correcting for unknown sample overlap.
Downloads
The software source is free to download and use from the author’s GitHub account.
Simulations are available in a separate repository.
Further information
You will find a simple introduction to the software in the README at the root of the bitbucket repository. Details on how the software behaves under various study designs can be found in the simulation repository.
This software is open source. You are free to fork and modify the software, provided you give credit to the original author.
If you make use of this software in your research please cite as follows : Southam L., Gilly A., Whole genome sequencing and imputation in isolated populations identify genetic associations with medically-relevant complex traits. Nat Commun. 2017 May 26;8:15606. doi: 10.1038/ncomms15606.
Contact
If you need help or have any queries, please contact us using the details below.
For all enquiries and questions, please contact Arthur Gilly directly.
Sanger Institute Contributors
Previous contributors
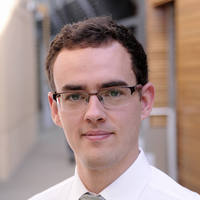
Arthur Gilly
Principal Bioinformatician
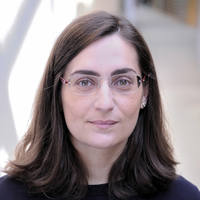
Dr Ioanna Tachmazidou
Staff Scientist