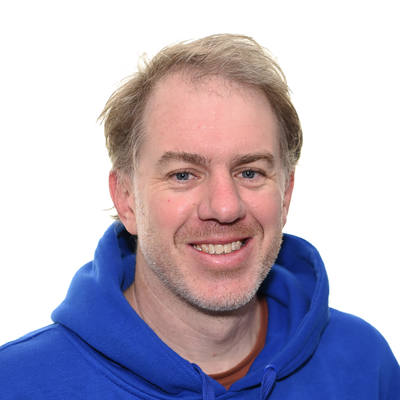
My main interest is in transforming biology into a quantitative and predictive engineering science. Despite 70 years of molecular biology and >20 years of genomics, we are still very limited in our ability to predict how biological systems respond to changes, even simple ones such as one or a few mutations. We are also embarrassingly limited in our ability to engineer biology. In short, I think the foundational problems of molecular biology – how amino acid and nucleotide sequences encode the properties of macromolecules – are still not sufficiently solved to be practically useful and that we need to focus on solving them.
Now is a really exciting time to be a biologist because we can finally generate the data required to directly tackle these foundational problems. Advances in DNA synthesis and sequencing allow us to perform hundreds of thousands of highly quantitative perturbation experiments at the same time. This is a remarkable opportunity and I often marvel that a student in my lab can perform a larger number of quantitative perturbation experiments than the whole world could 15 years ago.
Using large libraries of DNA variants, selection experiments and then DNA sequencing as a counter, we can precisely quantify how well millions of different proteins and RNAs perform different functions. Moreover, cheap computing and advances in artificial intelligence allow us to train predictive and mechanistic models from the data and to extract mechanistic understanding.
This opportunity means that not only can we build reference atlases of how changes in DNA sequence affect proteins and cause disease, but we can also use the data to train the predictive models needed to enable fast bioengineering. From protein stability to structure to aggregation to expression to binding affinities, we are no longer guessing in the dark but can test all the hypotheses at once!
I have always been interested in the biology of individuals – what makes individuals unique – and also in the challenge of making biology predictive. Over the years I have studied both how genetic variation causes phenotypic variation and why individuals still differ even when they are genetically identical.
My research has always involved both experimental and computational approaches and I have always tried to start from a question and then choose the best approach and method to address it. As such, my lab has worked with quite diverse methods and systems, including human genetic data (particularly cancer), model organisms (worms and yeast), large-scale genomics, small-scale quantitative experiments, mechanistic modelling and mechanism-free statistics and machine learning.
Over the years we have addressed some of the most basic questions in genetics, including why mutations have different outcomes in different individuals (incomplete penetrance and variable expressivity), how mutations interact, the rates and biases of mutation processes, dominance and dosage-sensitivity, and the importance of developmental noise and inter- and trans-generational epigenetic inheritance.
It was while I was a postdoctoral fellow at the Sanger Institute working with Andy Fraser that I became interested in understanding how mutations interact and using genetic interactions to understand biology. Back then we used RNAi to test the combined effects of tens of thousands of pairs of gene inhibitions for the first time in an animal.
In 2006 I started my own lab at the new EMBL-CRG Systems Biology Research Unit in Barcelona, where we initially mostly worked on phenotypic variation in isogenic individuals, before returning in a big way in recent years to quantifying what happens when thousands of different mutations are combined. At the CRG we were a cluster of quantitative groups using modelling, which has had a big impact on my thinking and we recently started the Barcelona Collaboratorium for Modelling and Predictive Biology to build further on this. At the Sanger Institute I want to combine this quantitative thinking with the ability to perform experiments at scale to help lay the foundations to make biology programmable.
The goal of my team and I is to produce models that allow researchers to understand, predict the action of, and engineer proteins so that they can produce the next wave therapeutics and drive forward bioengineering. Towards this goal, we are applying and developing our approaches to study all aspects of DNA sequence-to-activity encoding: including protein stability, allostery, protein-protein interactions, aggregation and RNA splicing.
I am proud of the fact that the work of my team is incredibly diverse, and that they are able to conduct their experiments at a scale that would previously have been impossible.
Everyone in my team has their own project and line of inquiry. I encourage independent thinking: I want my members to own their research and make the most of the resources available to them. Fifteen previous members of my lab are now principal investigators who run their own research groups. They are the most important achievement of the lab.
I am excited that my team and I are able to utilise the Sanger Institute’s resources to be able to run experiments that measure and test so many parameters of a protein’s generation and function. Before it was like working with a torch, now we can turn all the lights on.
My timeline
Senior Group Leader at the Wellcome Sanger Institute
Co-founder and co-chair, EMBL-CRG Barcelona Collaboratorium for Modelling and Predictive Biology
European Molecular Biology Organisation (EMBO) Member
Coordinator of Systems Biology Programme, CRG
EMBO Gold Medal
Liliane Bettencourt Prize for Life Sciences
The Genetics Society Balfour Prize
AXA Chair in risk prediction in age-related diseases, CRG
Senior Group Leader, Systems Biology Programme, CRG
Eppendorf Award for a Young European Investigator
The National Research Prize of Catalonia
Banc Sabadell Prize for Biomedical Research
The City of Barcelona Prize for Science
The FEBS Anniversary Prize
EMBO Young Investigator
Research Professor, Catalan Institute for Advanced Studies (ICREA)
Junior Group Leader, EMBL-CRG Systems Biology Research Unit
PhD, University of Cambridge
Postdoctoral Fellow, Wellcome Sanger Institute, UK
PhD student, MRC-HGMP, University of Cambridge
Mellon Scholar, Clare Hall College, University of Cambridge
BA, Natural Sciences (Biochemistry and Molecular Biology), First Class Honours, University of Cambridge
Hobbes Scholar, St Catharine’s College, University of Cambridge
The Biological Sciences Scholarship, St Catharine’s College
Visiting researcher, Cold Spring Harbor Laboratory, USA
The Natural Sciences Scholarship, St Catharine’s College