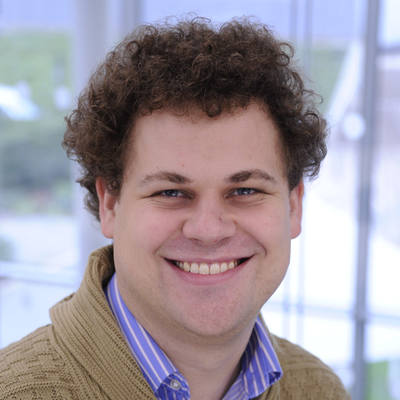
Alumni
This person is a member of Sanger Institute Alumni.
John Marioni is now Senior Vice President and Head of Computation at Genentech, San Francisco. You can keep up to date his latest publications at https://scholar.google.co.uk/citations?hl=en&user=oflcSSoAAAAJ
This page is no longer being updated and is a historical record of John Marioni’s work at the Sanger Institute.
John graduated from the University of Edinburgh in 2003 with a BSc in Mathematics and Statistics before obtaining an MPhil in Statistical Science at the University of Cambridge in 2004. Subsequently, he read for a PhD in the University of Cambridge, where he developed statistical approaches for analysing DNA copy number data. Upon completing his PhD in 2007 he moved to the University of Chicago, where he carried out post-doctoral research in the Department of Human Genetics. In Chicago, he focused on the analysis of RNA-sequencing data, developing novel methods that have become widely used in the field.
In 2010, he established his research group at the EMBL-European Bioinformatics Institute. His independent research has focused on understanding how differential gene expression is regulated between closely related species of mammals and in modelling variability in gene expression between single cells
John’s appointment in 2014 at the Sanger Institute enables his group to apply their computational approaches to novel biological questions through collaborations with faculty and research groups at the Institute.
My timeline
Senior Group Leader, CRUK Cambridge Institute, University of Cambridge
Associate Faculty, Wellcome Trust Sanger Institute
Research Group Leader, EMBL-EBI
University of Chicago, PostDoc, Human Genetics
University of Cambridge, PhD, Computational Biology
University of Cambridge, MPhil, Statistical Science
Graduate from University of Edinburgh, BSc in Maths and Statistics